Abstract
Although parallelism has been extensively used in Reinforcement Learning (RL), the quantitative effects of parallel exploration are not well understood theoretically. We study the benefits of simple parallel exploration for reward-free RL in linear Markov decision processes (MDPs) and two-player zero-sum Markov games (MGs). In contrast to the existing literature, which focuses on approaches that encourage agents to explore over a diverse set of policies, we show that using a single policy to guide exploration across all agents is sufficient to obtain an almost-linear speedup in all cases compared to their fully sequential counterpart. Furthermore, we demonstrate that this simple procedure is near-minimax optimal in the reward-free setting for linear MDPs. From a practical perspective, our paper shows that a single policy is sufficient and provably near-optimal for incorporating parallelism during the exploration phase.
Publication
International Conference on Artificial Intelligence and Statistics (AISTATS)
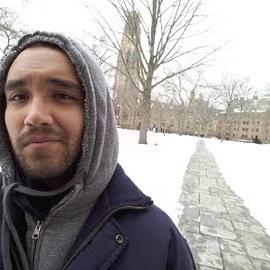
Pedro is a postdoctoral scholar and fellow at the University of Illinois, Urbana-Champaign (UIUC), affiliated to the department of Computer Science.
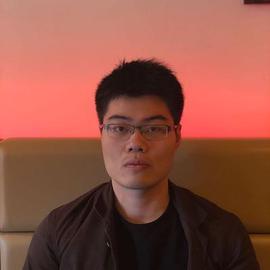
PhD Student
Boxiang is a PhD student in the Econometrics and Statistics dissertation area at University of Chicago Booth School of Business. Prior to coming to Booth, he obtained a Master of Science in Machine Learning (2019) and a Bachelor of Science in Statistics and Machine Learning (2018) from Carnegie Mellon University.
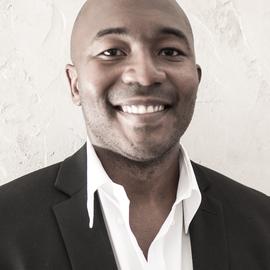
Sanmi Koyejo an Assistant Professor in the Department of Computer Science at Stanford University. Koyejo’s research interests are in developing the principles and practice of trustworthy machine learning. Additionally, Koyejo focuses on applications to neuroscience and healthcare. Koyejo has been the recipient of several awards, including a best paper award from the conference on uncertainty in artificial intelligence (UAI), a Skip Ellis Early Career Award, and a Sloan Fellowship.
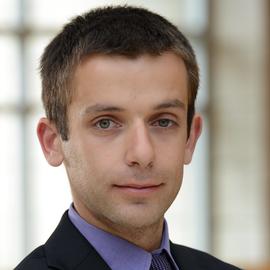
Associate Professor of Econometrics and Statistics
Mladen Kolar is an Associate Professor of Econometrics and Statistics at the University of Chicago Booth School of Business. His research is focused on high-dimensional statistical methods, graphical models, varying-coefficient models and data mining, driven by the need to uncover interesting and scientifically meaningful structures from observational data.